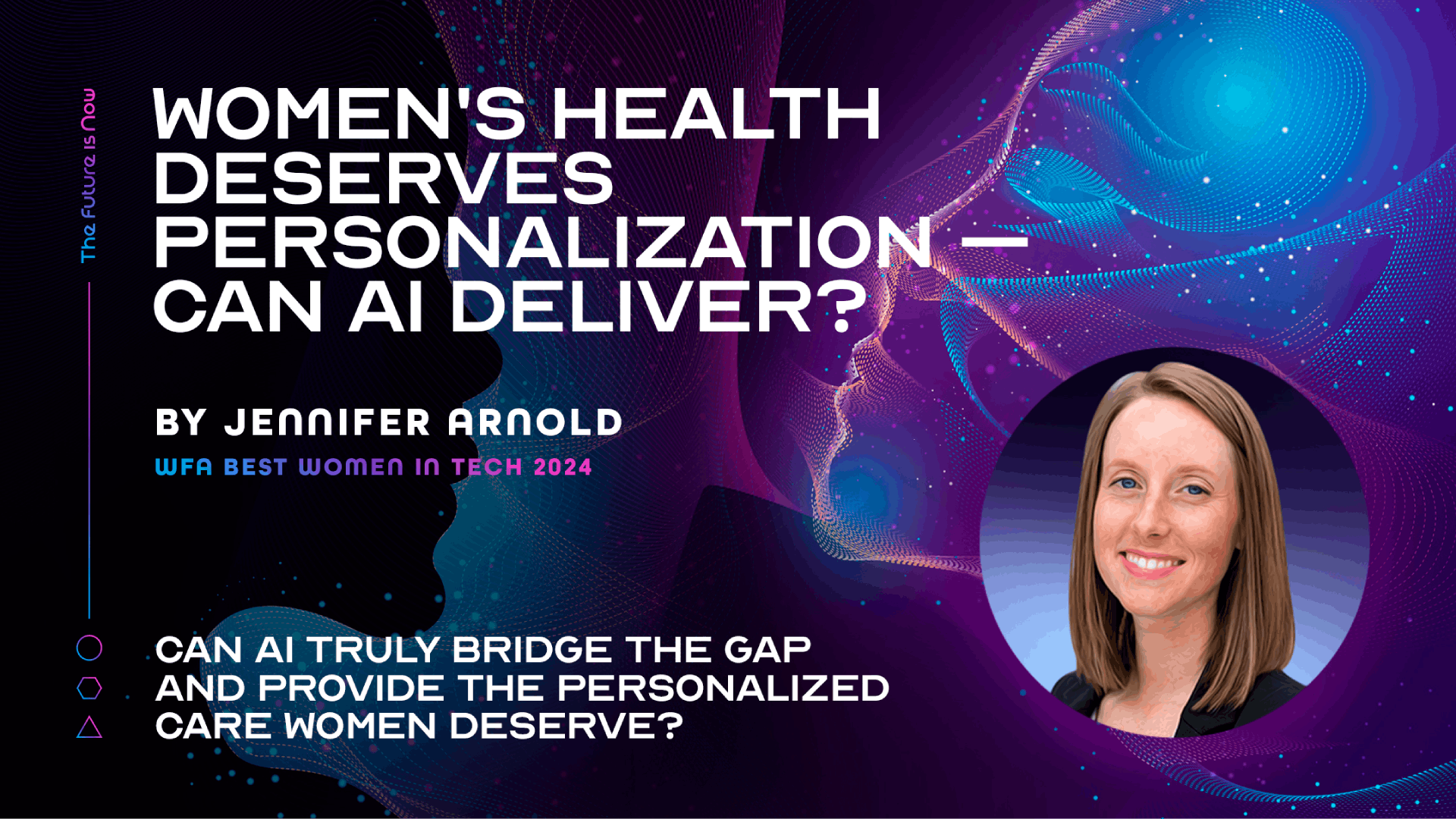
Women’s Health Deserves Personalization – Can AI Deliver?
By Jennifer Arnold, Engineer | Healthcare Startup Executive | Women’s Health Advocate
Women’s healthcare has long relied on standardized protocols, a disservice to the nuanced needs of female physiology. Women drive 80% of healthcare decisions, yet 59% feel their needs are unmet1, revealing a market and clinical failure. Artificial intelligence (AI)—a probabilistic system that can learn from data and improve over time2—offers unprecedented potential to close this gap. But can these smart technologies truly deliver the personalized care women deserve?
How AI Makes Personalization Possible
AI’s power in women’s health lies in its ability to process vast, diverse data simultaneously. Modern neural networks—computational systems inspired by human brain connections—analyze hormonal patterns, genetics, symptoms, and behaviors, creating comprehensive models beyond traditional methods3. For endometriosis, where diagnosis averages 7-11 years4, AI systems show remarkable accuracy in early detection by finding subtle connections doctors might miss during brief appointments. Specifically, a study published in the Journal of Biomedical Informatics demonstrated that machine learning algorithms, when trained on patient-reported symptoms and medical history, could identify women at high risk for endometriosis with an accuracy of 89%5, helping reduce diagnostic delays. This means AI can sift through the complex web of symptoms, medical history, and even genetic predispositions to flag at-risk individuals potentially earlier than traditional methods.
What makes these systems particularly valuable is their ability to recognize patterns over time. Recurrent neural networks (RNNs), a specialized type of neural network, function like digital memory systems that remember past information, enabling them to track how female physiology changes through menstrual cycles, pregnancy, perimenopause, and beyond. Unlike standard neural networks that process each input independently, RNNs use feedback loops to give them a ‘memory’ of previous inputs, which is crucial for understanding sequential data3. Imagine an AI system that can analyze years’ worth of menstrual cycle data to predict hormonal imbalances or identify early signs of ovarian disorders. This addresses a fundamental limitation in traditional medicine: the tendency to take isolated snapshots rather than analyzing the dynamic, cyclical nature of women’s health.
Building on Limited Research Through Smart Computing
Historically, women were excluded from clinical trials, creating knowledge gaps. Before 1993, when FDA guidelines changed, women of childbearing age were routinely excluded from drug studies6—resulting in treatments optimized for male physiology. AI offers a revolutionary solution: extracting meaningful insights even when female participants are a fraction of study populations.
Through a technique called “data augmentation,” AI can artificially expand limited female datasets7. Imagine having data from only 100 women in a clinical trial of 1,000 participants. AI can analyze these 100 cases intensively, identifying subtle patterns and generating synthetic data points that represent similar women with slightly varied characteristics. This computational approach effectively “amplifies” the female voice in historical research without fabricating results. It is important to note that data augmentation is well-suited for predictive inference. However, these methods are not appropriate for determining the causal effect of the drug on a subpopulation of women. Data augmentation is best suited for prediction (what will happen) rather than causal analysis (why it will happen)8.
Similarly, “domain adaptation” algorithms can identify which insights from male-dominated studies might safely apply to women and which require female-specific recalibration. For example, an AI might recognize that a certain heart medication dosage needs adjustment for women due to physiological differences in metabolism and body weight9.
Modern AI performs post-hoc analysis on published studies, extracting female-specific data that may have been collected but never separately analyzed. For example, by reanalyzing historical medical records and tracking treatments and responses over time, AI can reveal insights about medication dosing, side effect profiles, and treatment efficacy for women that have been hidden. A study published in The Lancet in 2022 highlighted how retrospective analysis of cardiovascular trials revealed significant differences in drug response and adverse effects between men and women, insights that were previously overlooked due to aggregated data10. This computational archaeology reveals that many women have been consistently over-medicated or undertreated based on male-derived standards—problems AI can now help correct.
Ensuring Responsible Innovation
Despite its promise, AI in women’s health faces substantial challenges. Algorithmic bias remains pervasive—studies have shown that many healthcare algorithms underperform for racial and ethnic minorities due to biased training data. For example, A study revealed that AI tools used to diagnose asymptomatic bacterial vaginosis exhibited ethnic disparities11. This is because AI models learn from the data they are trained on, and if that data reflects existing biases, the AI will perpetuate them. Addressing this requires diverse development teams and training data, alongside rigorous validation across demographic groups.
Privacy concerns are equally critical given the sensitive nature of reproductive health data. Advanced protection techniques, such as differential privacy and federated learning, can safeguard individual information while preserving analytical insights12.
The Path Forward
As the femtech sector expands toward a projected $43 billion by 203413, AI stands poised to revolutionize women’s healthcare, offering a powerful tool to address systemic disparities. By leveraging AI’s capacity, we can uncover insights that have eluded traditional research methods, potentially saving millions of lives and billions in healthcare costs.
However, realizing this potential hinges on ethical implementation and inclusive design. As we stand at this technological crossroads, it is imperative that we approach AI development in women’s health with a focus on equity, transparency, and accountability. The power of AI to transform women’s healthcare is undeniable, but its impact—whether it narrows or widens health disparities—ultimately depends on how we choose to wield this powerful tool. By prioritizing diverse representation in AI development and rigorously addressing potential biases, we can ensure that this technological revolution truly serves all women, marking a new era of personalized, effective, and accessible healthcare.
References:
1. Lux Capital. (2025). Unlocking Potential: Artificial Intelligence for Women’s Health.
2. IBM. (2024). What is Artificial Intelligence (AI)?
3. Aledade. (2024). AI in Healthcare: Improving Patient Outcomes.
4. WomenTech Network. (2024). What Role Does AI Play in Advancing Women’s Health Initiatives?
5. Journal of Biomedical Informatics. (2023). Machine learning for early detection of endometriosis: A prospective study.
6. Harvard Business Review. (2024). How AI Could Help Reduce Inequities in Health Care.
7. Shorten, C., Khoshgoftaar, T.M. (2019). A survey on Image Data Augmentation for Deep Learning. Journal of Big Data 6, 60.
8. Pearl, J., Glymour, M., Jewell, N.P. (2016). Causal Inference in Statistics: A Primer. John Wiley & Sons.
9. Franconi, F., & Campesi, I. (2014). Sex and gender differences in pharmacology. British journal of pharmacology, 171(10), 2294–2308.
10. Lancet, The. (2022). Sex differences in cardiovascular disease. 399(10333), 1389-1400.
11. University of Florida. (2023, November 20). Study reveals bias in AI tools when diagnosing women’s health issue. ScienceDaily.
12. OpenMined. (2024). What is Federated Learning?
13. GreenBook. (2024). The Femtech Frontier: Embracing AI for Women’s Health in 2024.
MORE NEWS
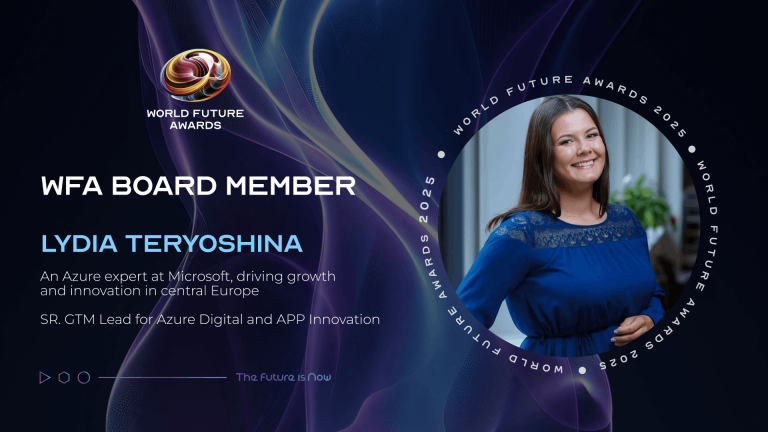
Lydia Teryoshina Appointed to World Future Awards Board of Experts
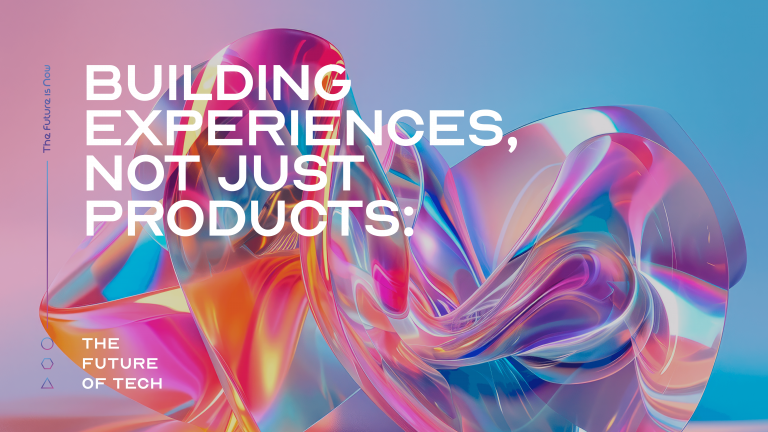
Building Experiences, Not Just Products: The Future of Tech
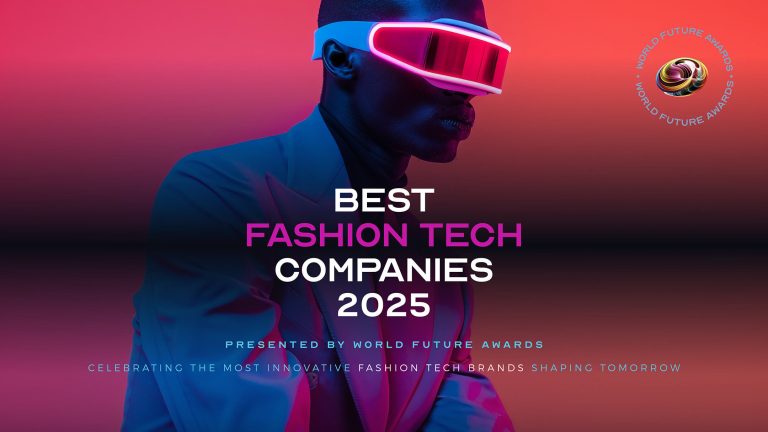
🌐 World Future Awards Honors the Best Fashion Tech Companies in the World 2025
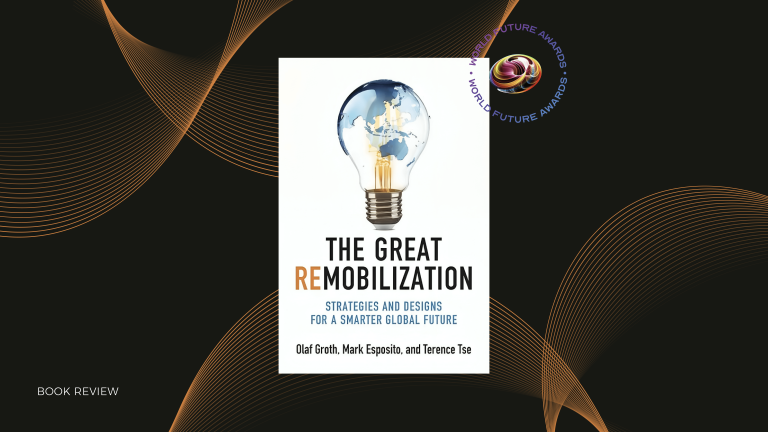
Book Review: The Great Remobilization: Strategies and Designs for a Smarter Global Future, by Mark Esposito
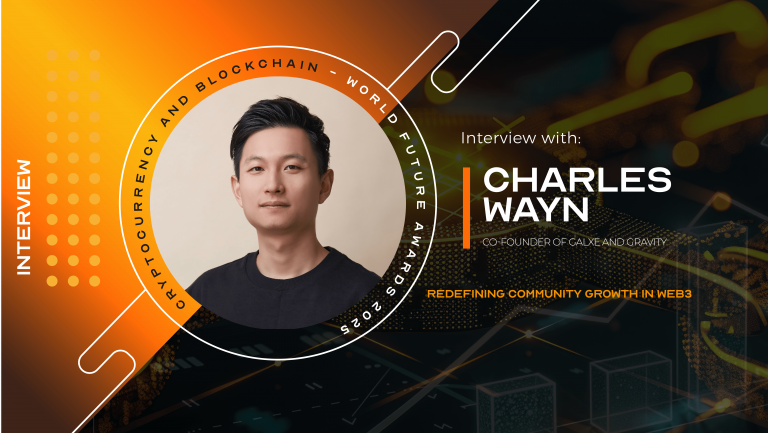
Redefining Community Growth in Web3: An Interview with Charles Wayn, Co-Founder of Galxe and Gravity
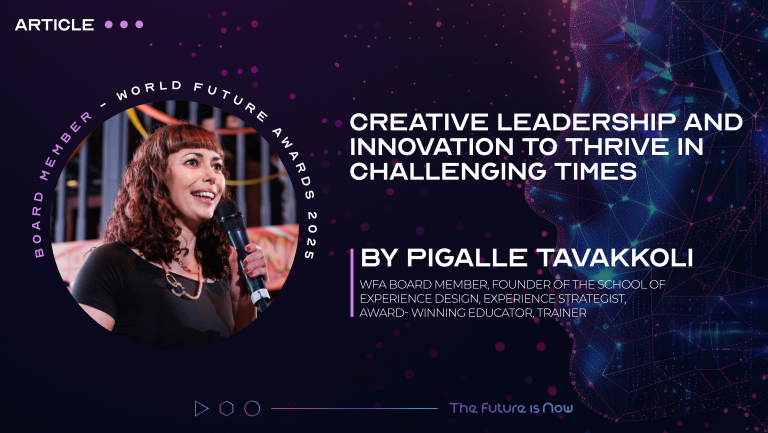
Creative Leadership and Innovation to Thrive in Challenging Times
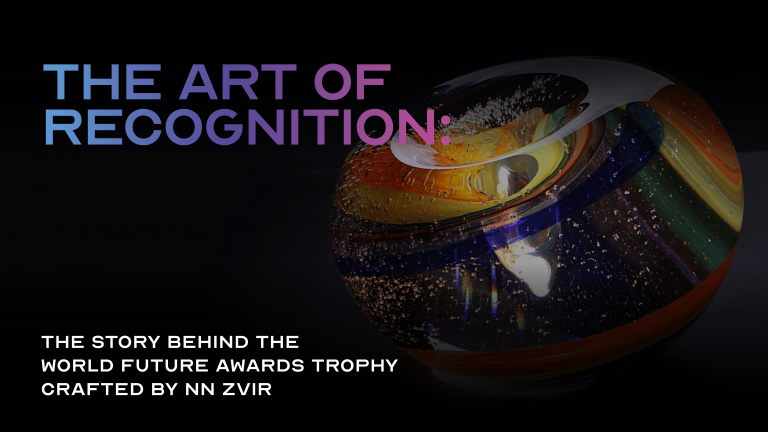
The Art of Recognition: The Story Behind the World Future Awards Trophy Crafted by NN ZVIR
NEWSLETTER
Sign up to learn more about our project and to stay up to date.